Airdropping Tokens to Users using a Community-Specific Reputation Score (Mirror.xyz)
Using graph algorithms to identify how to grow your community through token distributions - full github code linked.
This article covers the methodology behind a community based reputation score, and then also the code behind the $WRITE token airdrop in 2021.
In my previous post on digital identity, I mentioned that "the tokenization of these graph shards could take many forms and will likely be layered upon by proof tokens." I believe that sharded graph identity approach requires coming up with a community-specific reputation score that measures how influential a certain person has been in expanding a specific network. While some reputation scores may be more set in terms of having to have done X or Y actions, this score captures a users reputation in the context of other users in a more fluid manner - acting like a signal rather than a badge.
Typically in Web2, users are "rewarded" by an algorithm which will highlight them based on the engagement and attention they bring to the platform. Thus, their reputation score is just the number of likes or followers they have - regardless of who those vanity metrics come from. In Web3, we typically reward with tokens representing the value of the protocol or product. These tokens also carry a lot of power in the form of voting and other privileges. As such, a score that signals not only influence but also how supportive or aligned a user is with the rest of the community will become increasingly important over time.
For this post, we will be focusing on creating a reputation score for Mirror users (voters, writers, contributors) based on where each user sits on an interaction-based social graph across Ethereum, Twitter, and Mirror data. I chose Mirror for three reasons:
I'm already very familiar with their community and product
They have verifiable consolidation of identity on their platform (Ethereum <> Twitter), allowing me to layer social graphs together.
Their product and governance are heavily user-to-user interaction focused, something most products on Ethereum don't currently have (we mostly interact with pools or marketplace protocols).
Social graphs themselves are not new, but selectively layering them will open up quite a few new doors of usability and meaningfulness. There are two main reasons for this:
Enabling new applications: We can use this graph data to create a context-dependent community-specific reputation score, which is then applicable in many situations.
What the data represents: The creation of a representational network built off of scarcity creates a social graph that proxies how users support one another.
Enabling new applications: The way that we understand the layering affects what we do with the data. The way I see it, Ethereum is a base layer social graph that everything else is built upon. Platforms like Mirror and Twitter are contexts that sit on top of this base layer and shift how we see the connection of users across the space.
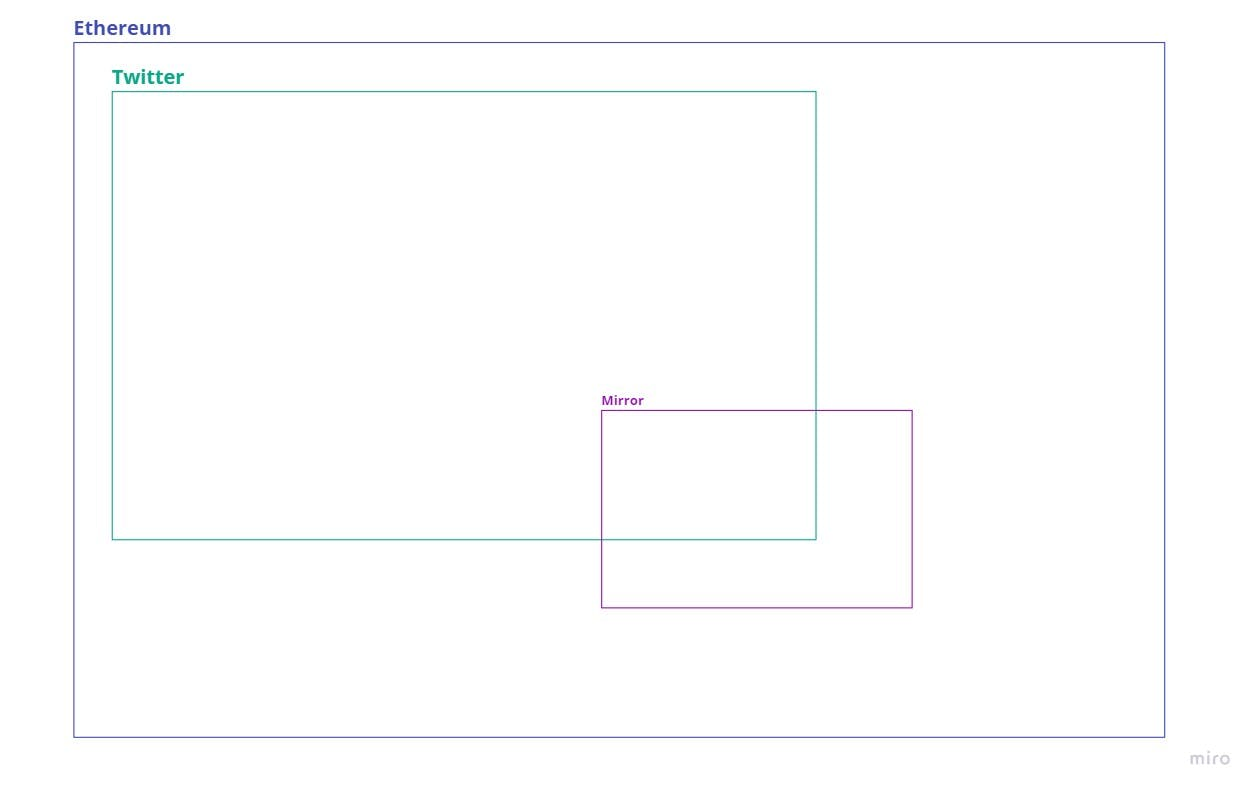
Because I wanted to analyze only users of Mirror, I took just a subset of available Ethereum and Twitter data. Those who have a high community-specific reputation score could get a larger token airdrop, be delegated for roles (or more votes) in treasury management, or get preferential access to new protocol features. There are also many more such contexts like Mirror built on top of Web2 + Web3 platforms, such as NFT communities (Cryptopunks, BAYC, Blitmaps) and gaming communities (Axie Infinity, Darkforest).
As DAOs start working together more and the metaverse becomes more interconnected, we'll see more communities (and contexts) overlap. I imagine that studying how people and communities interact within and across different contexts of the social graph could produce mixed-community reputation scores which could be applied in quite a few different situations. For example, this score could be used for multi-token or collaborative NFT airdrops, as well as choosing leaders for partnerships and programs like rabbithole's pathfinder initiative.
What the data represents: The kinds of data collected and used affect the social graph as well. Using data from $WRITE votes, funding contributions (across editions, splits, crowdfunds, and auctions), and Twitter mentions, I want to represent three buckets of scarcity (respectively): belief, capital, and attention.
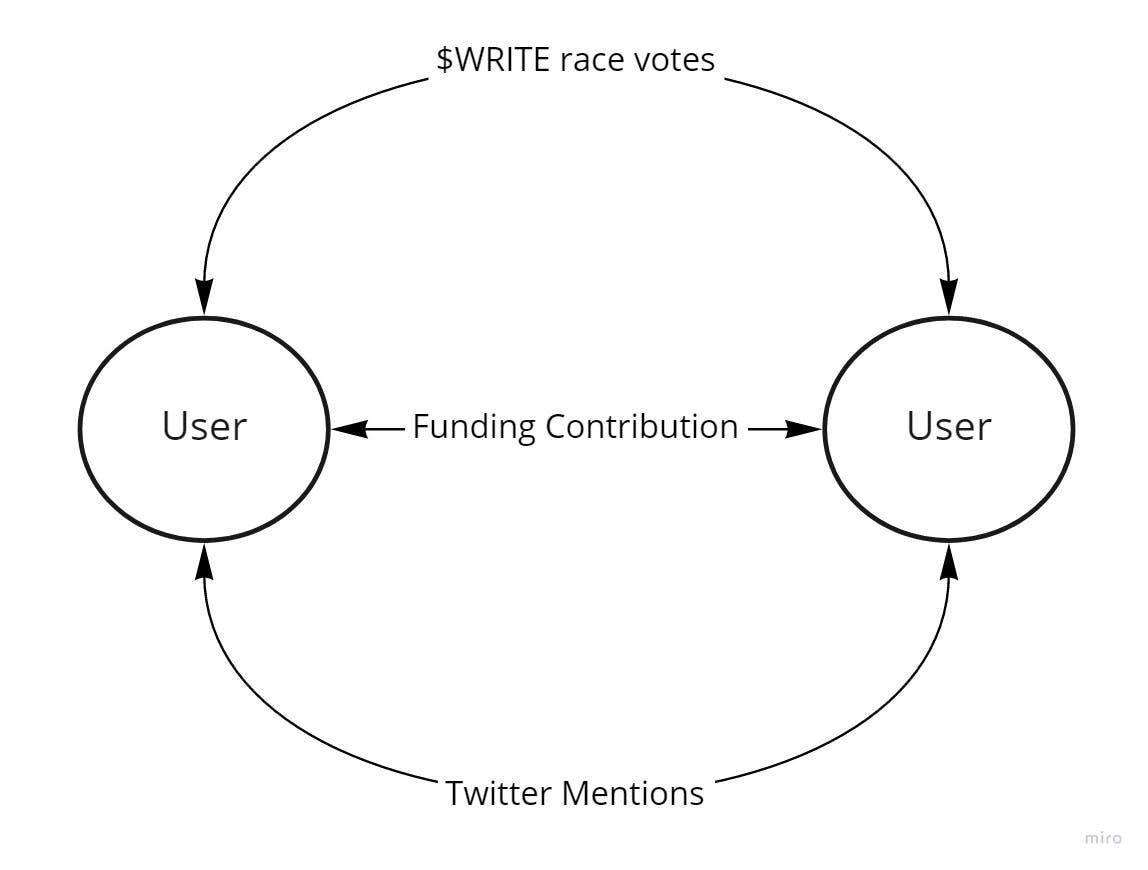
I believe that analyzing how interactions connect different people across these three buckets gives us a proxy for how much they support each other. I also believe that networks of support give us a more accurate representation of communities within social graphs. These two assumptions gave me confidence in using a concept called "betweenness centrality" as a primitive for a reputation score.
The selection of data representation and contexts was key to the form the social graph ultimately took. If I wanted to proxy just willingness to contribute, then I would probably create a completely create node schemas based on different kinds of products/categories of creators rather than a pure user-to-user graph. This would likely shift the shape of the social graph completely.
Using Graph Data Science to Create a Community Reputation Score: Betweenness Centrality
Let's try to target and quantify the strong connection points of the social graph of race participants, writers, and contributors. I've chosen to use a concept called "betweenness centrality" to represent the score of each node. Betweenness centrality is calculated using an algorithm that calculates the shortest path between all nodes for all nodes).
Community clusters in a social graph usually look something like this:
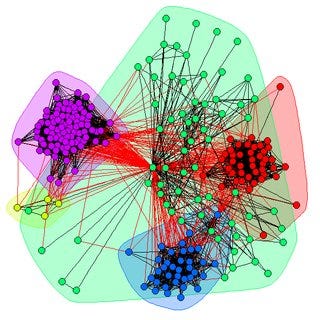
For Mirror, the base social graph of who has voted for who looks like this:
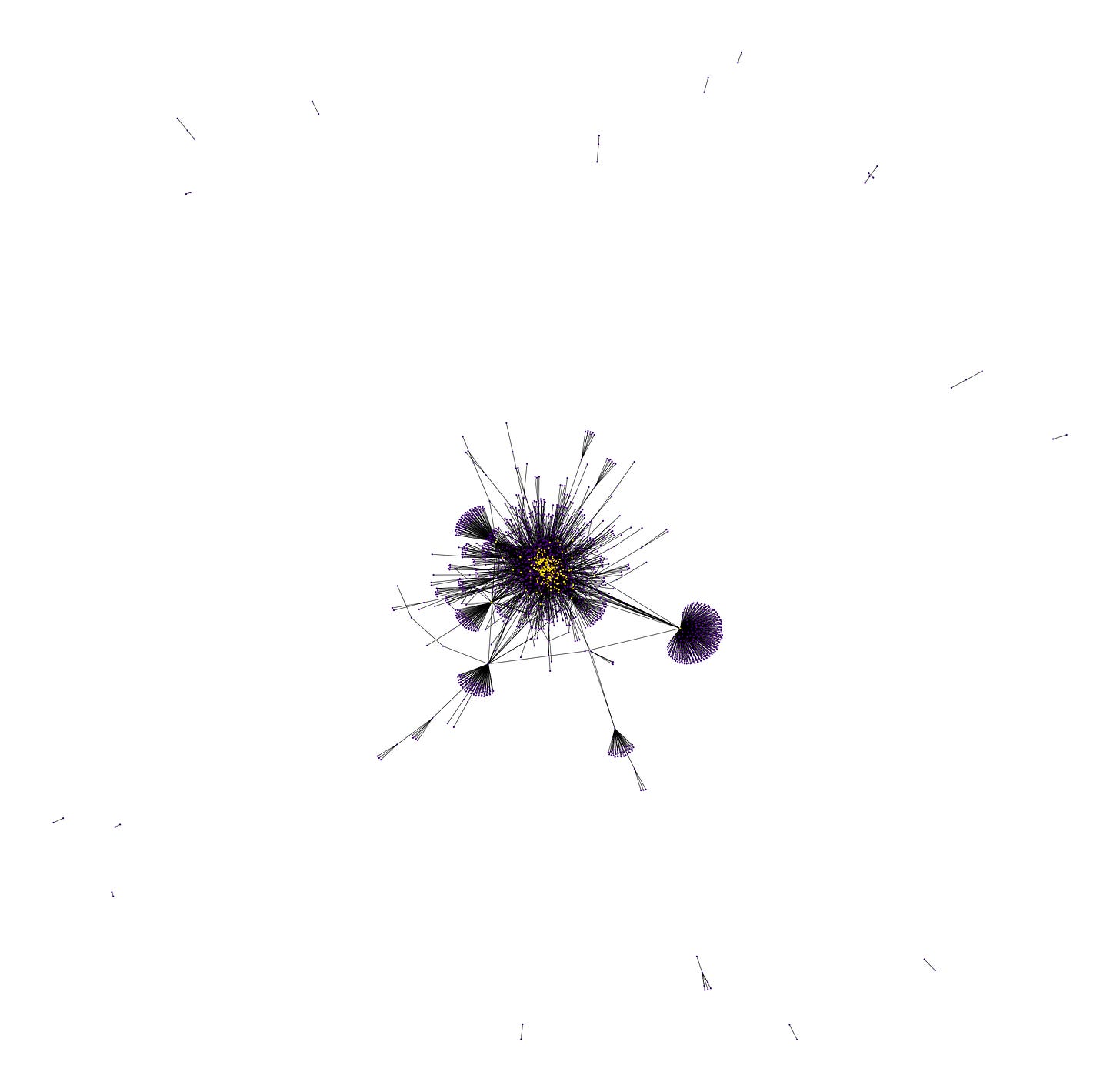
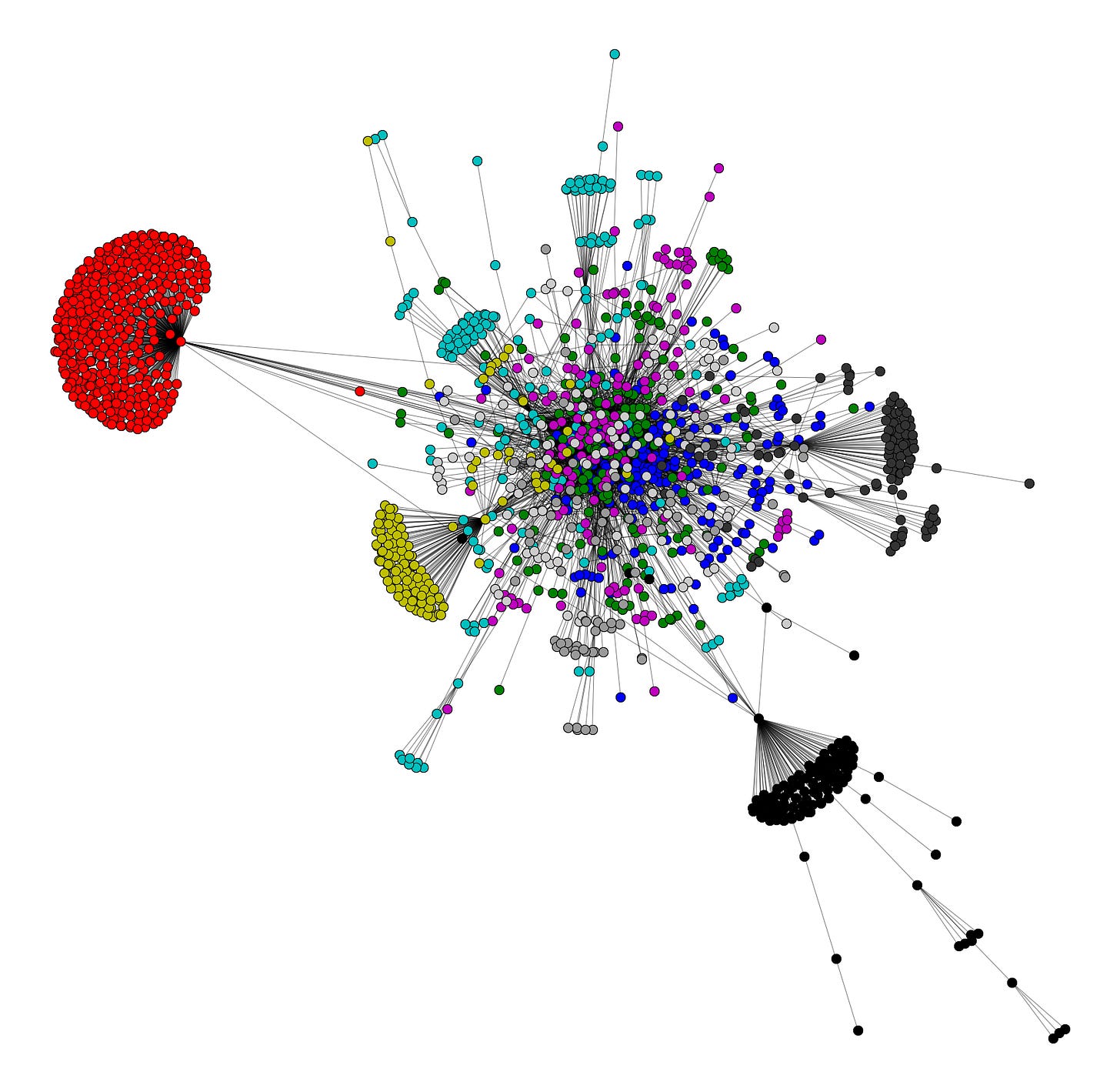
However, this doesn't have an outside-of-race social context yet. Let's layer in Ethereum transaction-level data - this is limited to Mirror-related transactions between participants, such as sending a split, funding a crowdfund, and buying an edition or reserve auction.
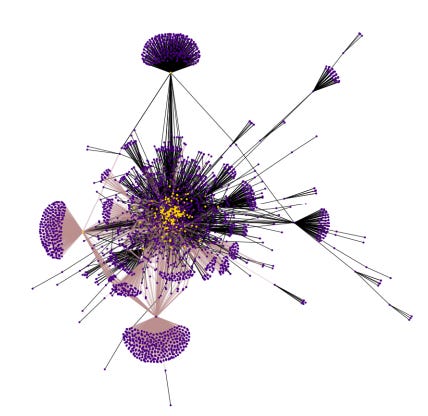
Now we'll add Twitter data too, which will link nodes based on who has mentioned another participant in their last 2000 tweets.
Showing the interactions (edges) in a cleaner fashion, the mix of interactions across the social graph looks like this:
Now getting back to the original point, the expectation is that some of these nodes between large clusters would get a higher weighting than others since they've connected the paths across the most other nodes.
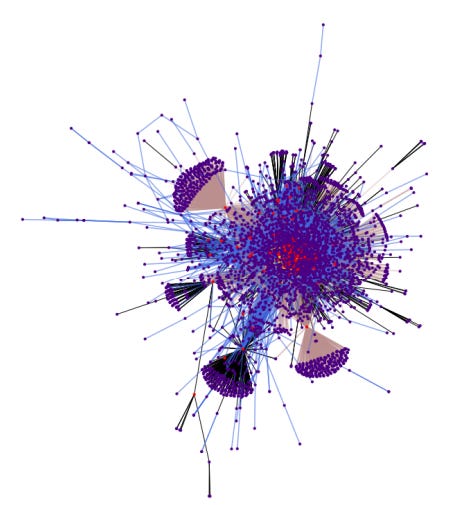
This is called "betweenness" and is a factor I believe to be very important when trying to proactively grow a community. The base idea here is that the higher the "betweenness" factor a person has, the more likely they will lead to creating connections and branches that build up a more diverse community. There have been a few research papers that highlight the beneficial effect that nodes with high betweenness have on the diffusion of a community as well as building the resilience of a network.
Some of you might be wondering why I chose betweenness centrality over something like closeness or degree centrality. Those latter two metrics highlight pure influence, and I don't think reputation in a community should be based on just those who already have that level of influence. The Graph Algorithms textbook by Neo4j puts the concept behind betweenness very well:
"Sometimes the most important cog in the system is not the one with the most overt power or the highest status. Sometimes it’s the middlemen that connect groups or the brokers who have the most control over resources or the flow of information. Betweenness Centrality is a way of detecting the amount of influence a node has over the flow of information or resources in a graph. It is typically used to find nodes that serve as a bridge from one part of a graph to another."
A lot of Web2 has been about concentrated influence and echo chambers, I believe Web3 should try to enable and incentivize creating bridges as much as possible instead.
For anyone who wants to see the scores, check out the sheet here. Note that these values have not been weighted based on data/edge type, so some users may have higher betweenness due to Twitter interactions rather than Mirror/Ethereum interactions.
Why is this Methodology Important to the Score?
We've already discussed some of the applications of this score, but the solution I used may still appear unnecessarily complex or overengineered. I think this methodology is required to provide composability while also measuring reputation in a way that is much tougher to game.
Composability: As mentioned before, this graph is built up layer-by-layer. The data elements are all publicly available to collect, the model follows a search algorithm and is flexible to whatever nodes or edges (users or interactions) are chosen, and once the pipeline is built once it can be reused or forked for any set of tweaks. Hopefully in the future, all the available data components just sit in a user interface and this model becomes drag and drop. From there I imagine you could export scores or connect directly to something like disperse.app or galaxy drops.
Durability: The problem with many scores and measures is that once they have been publicly used once, then people can start to figure out how to game the system. This is especially true for anything that is just user <> protocol
interaction based. Creating a model that is user <> user <> user
dependent is harder to game because the users in the current community won't necessarily reciprocate interactions with a bad actor. Actions are also compounding, so having just one interaction (or one type of interaction) will not be good enough to get a higher score). Even if they do find some way to game it, then congratulations you now have another active participant who is contributing to the community.
These two elements allow for an overall stronger reputation score mechanism, which I believe justifies the efforts it takes to get there.
A (very) short history on token airdrops:
Airdrops have come a long way in the last year, most notably starting from Uniswap's 400 UNI token airdrop in September of 2020. From there, we've grown into airdrops that are calculated based on the frequency and volume of interactions with different facets of a protocol. Below is one such example:
We've also seen NFT drops recently that rely on more standardized tools built for quickly setting rules/requirements and rewards/distribution. None of these methodologies are bad, but I do believe there's still a lot of room for improvement. This could come from the distribution model, drop frequency, or even the token rules themselves. In this proposal, I'll be focusing on a new distribution model, one that combines data from user interactions in the Mirror $WRITE race, on Twitter, and in Mirror-related Ethereum transactions.
Rewards, Incentives, and Governance:
The Mirror $WRITE race is a vote-based competition that occurs once a week, where the top ten writers get 1 $WRITE token. This gives them access to the platform as well as 1000 votes for the future rounds of the race. The structure is not dissimilar from most Sybil-resistant approaches where a validated party votes in a new party, however, the propagation of rounds is a lot slower as it only happens once a week. This means it takes a lot longer to really diversify away from the friends/communities of the genesis writers of the $WRITE race. For new participants who aren't very close to that initial circle, it becomes harder and harder to win as the top 10 vote floor continues to rise:
While increasing the # of winners and base vote allocation or gamifying the prompt system could incrementally improve the race, an airdrop of $WRITE tokens would offer a much faster method of reducing the influence of the starter group and increase the diversity of writers (winners) on the platform.
A key first decision here is that airdropped tokens should not affect the current vote allocation for each participant in the $WRITE race. But, they can still be redeemed for publications**, at 1 $WRITE token per publication.
My first thoughts when structuring this airdrop model were around "what should an airdrop really be targeting?" The three pillars that came to mind were:
Rewards: How can we fairly compensate early users for their support and risks that were taken? They chose to publish on Mirror rather than their existing publishing platform, carrying both new funnel and smart contract risks.
Incentives: Knowing that this model will set a precedent for rewards distribution criteria, how will that affect voter/creator/contributor behaviors going forward?
Governance: How can the balance of power be shifted in a way that diversifies the writer community much faster?
From there, I set three goals for the token formula:
Rewards: Creators should be rewarded for their usage of web3 platform features, and contributors should be rewarded for the value (ETH) they've provided to creator projects
Incentives: We should also incentivize contributors to support a larger array of creators, rather than just the same two or three creators (or a single community).
Governance: We should try to amplify anyone who bridges different communities by giving them more tokens.
We know that for Mirror to thrive long-term, there needs to be diversity in creators with an equally diverse set of contributors. However, with over 7000 users it can be hard to choose who gets what allocation off of usage alone. This is where the social graphs enter the picture, as we can use that data to identify who might be best from a community perspective to allocate greater or fewer airdrop tokens to.
Token Reward Formula:
For all of the following, $W$ stands for a weight between 0-1 and $T$ stands for $WRITE token.
First, let's take care of the community diversification weighting based on centrality:
Betweenness centrality is a measure that highlights the nodes that bridge the most communities. This serves to incentivize everyone to support and collaborate with more diverse communities in the future. See this post for details on this metric and the methodology. We multiply it by 50 to give it more weight.
While the linked post above does not account for the difference in edge/relationship data type, so a mention and a 1 ETH contribution would have the same non-scaled weight), for this airdrop, we converted the multi-edge graph into a weighted directional graph. This means normalizing each edge by total mentions/contributions/votes a certain user has given, and then weighting mentions, contributions, and votes by 15x, 40x, 40x respectively. Another important change from the original post is the use of a directional graph.
We still want to make sure creators and contributors are getting fairly rewarded. Let's define the T_baseReward formula as:
V_votesAllocated represents the current allocation of votes someone has in the $WRITE race. This is divided by 1000 to represent the $WRITE token balance. This is weighted by W_voted which represents the total votes used out of votes allocated each week (cumulatively).
B_hasCreated represents a Boolean (1 or 0 value) for if someone has created at least one entry or block (edition, split, crowdfund, auction).
V^n_contributed is the value of ETH contributed to creators (across n different block types). This is weighted by the unique # of creators someone has contributed to, max_min scaled into W_contributed. This means if the maximum number of unique creators someone has contributed to is 10, and I've also contributed to 10 creators, then I would have a W_contributed of 1. Depending on the distribution of contributors, if I've only contributed to 1 creator, I would have a W_contributed of something like 0.0833. Thus, we are rewarding those who both contribute to creators and support many creators, in an effort to incentivize that behavior.
The constants of 0.65 and 0.15 were chosen based on how many total tokens we wanted to distribute.
Putting it all together, we get our total $WRITE token reward formula:
Here is the github with all the scripts, and here is the data dictionary.
What a Reputation-based Airdrop Enables for the Community:
I believe this airdrop has iterated upon everything we built in web2 and web3, mainly due to its combined use of multiple social graphs alongside tokenized ownership. As I've covered before, betweenness centrality is a measure that helps us target a more resilient and diverse network. Tokens are a great way to reward those who have fulfilled that behavior historically, and also as an incentive mechanism to further that diversity and resilience (support) in the future.
This proposal indirectly tackles the issue of long-term ownership of tokens, where many receivers (other than core investors and the product team) sell the tokens soon after the drop. My hope is that if the tokens are distributed to an aligned subset of the community, we won't see this issue appear as strongly. I think the Ohmies are a great example of this done well.
While this airdrop is at a protocol level, there's no reason why we couldn't go more micro in our approach and provide creators and DAOs betweenness scores for their specific social graph of contributors, voters, and Twitter interactors. They could leverage that data to then airdrop their own tokens as well. Ultimately, I think that what has been done here can be further expanded and productized to help all of us gain better visibility into and reward/incentivize each step of the community flywheel created by the team at 0xSTATION:
Sources and Methods:
All data (besides identity mappings) were publicly sourced. Please see the data dictionary for details on sources.
Data analysis and visualization were performed in Python, using a mix of Pandas, Numpy, Scikit-learn, Networkx, Matplotlib, Seaborn, Plotly, Cdlib, Gephi, and Neo4j. The Neo4j Dashboard, Bloom, and Graph Data Science (GDS) packages were used as well for ancillary analysis. Desmos was used for early exploration and tuning of the reward formula.